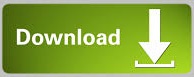
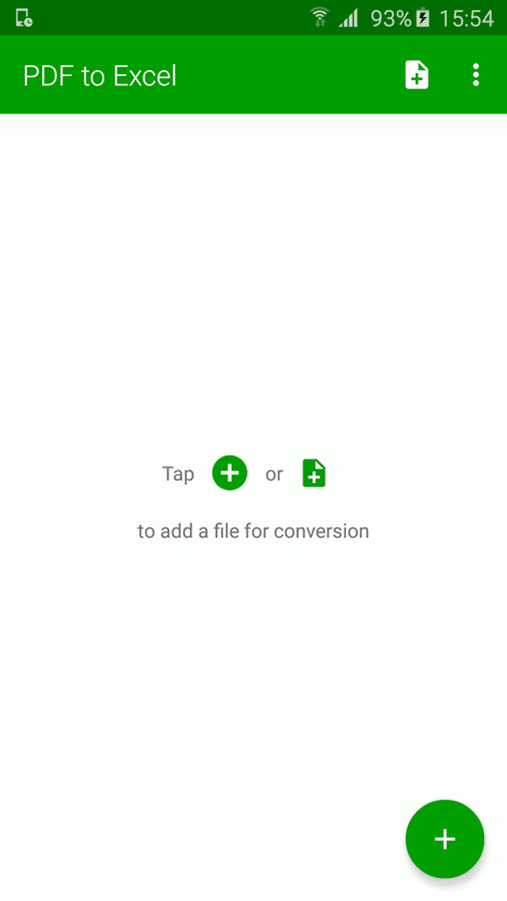
Wilks lambda: The results given by minimizing this criterion are identical to that given by the determinant of W. Furthermore, group sizes may be less homogeneous than with the trace criterion. Moreover, this criterion tends to produce classes of the same size.ĭeterminant(W): The determinant of W, pooled within covariance matrix, is a criterion considerably less sensitive to effects of scale than the W trace criterion. In order to avoid giving more weight to certain variables and not to others, the data must be normalized beforehand. This criterion is sensitive to effects of scale. Minimizing the W trace for a given number of classes amounts to minimizing the total within-class variance - in other words, minimizing the heterogeneity of the groups. Trace(W): The W trace, pooled SSCP matrix, is the most traditional criterion. XLSTAT offers four criteria for the k-means minimization algorithm: Several classification criteria may be used to reach a solution. Classification criteria for k-means Clustering
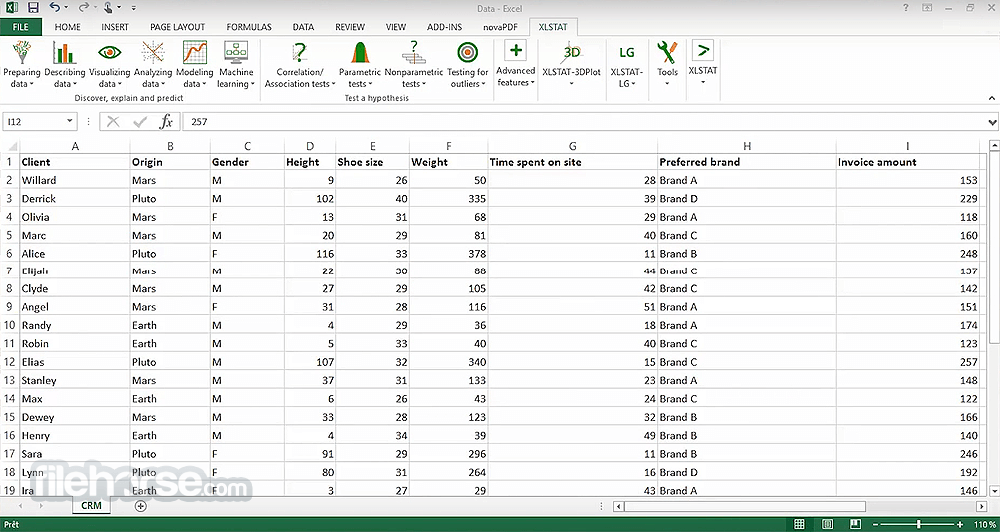
The objects are then reassigned depending on their distances from the new centers. Then the centers are redefined from the objects assigned to the various classes. Afterwards, the distance between the objects and the k centers are calculated, and the objects are assigned to the centers they are nearest to. For this reason, the calculations are generally repeated several times in order to choose the optimal solution for the selected criterion.įor the first iteration, a starting point is chosen which consists of associating the center of the k classes with k objects (either taken at random or not). The solution obtained is not necessarily the same for all starting points.

K-means clustering is an iterative method which, wherever it starts from, converges on a solution. Note: if you want to take qualitative variables into account in the clustering, you must first perform a Multiple Correspondence Analysis (MCA) and consider the resulting coordinates of the observations on the factorial axes as new variables. The k-means and AHC methods are therefore complementary. The disadvantage of this method is that it does not give a consistent number of classes or enable the proximity between classes or objects to be determined.
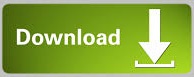